AI is the buzz word in technology these days and everyone seems to be using it (or misusing - a recent edtech ad). Despite the hype, AI is here to stay and more importantly grow. And the growth is not going to be small by any means and measure. While AI is a 1000 ft view, there are multiple technologies that are growing pretty quickly under that umbrella – Machine Learning, Deep Learning, GANs etc.
Multiple industries and domains have multiple use cases for AI implementation. But in this article, we shall be focusing on one domain – Health care.
In Health care we are going to witness a wide adoption of AI with wide variety of applications in multiple areas like Prognosis, Diagnosis and Treatment. Let’s look at each of these three areas at a high level first and then we shall discuss about some use cases in the Diagnosis of Conditions.
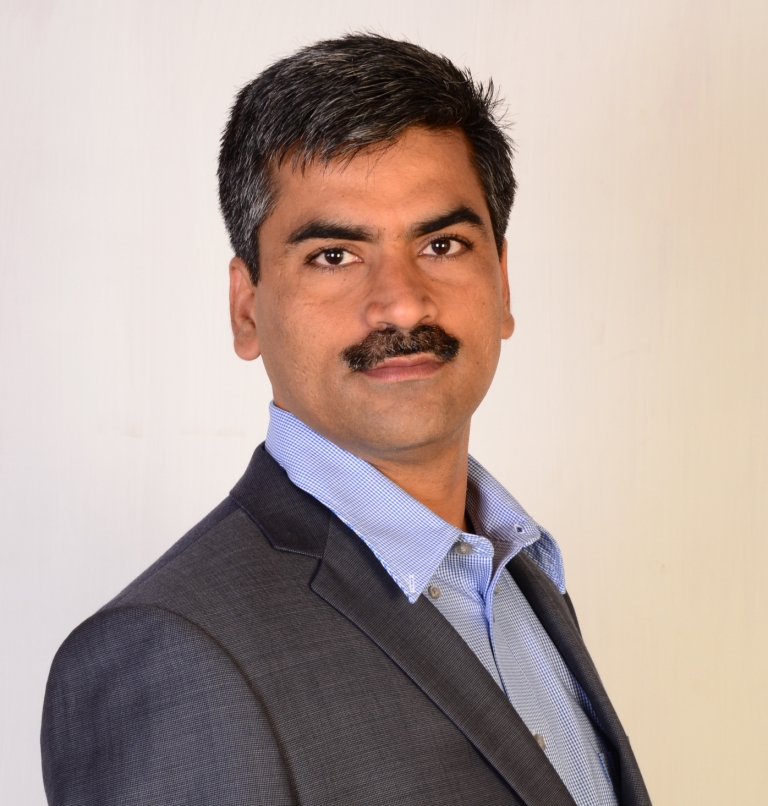
Prognosis – Prognosis involves the prediction of certain conditions based on certain statistical data. Given the complexity of the human body, it is not easy to predict future conditions. Medical practitioners and the data custodians can come out with some rules by taking few parameters into consideration to come out with predictions and outcomes. But that will be quite limited and that’s where Deep Learning can help. If the AI model can be trained with quite a bit of data with multiple parameters, it can come out with some outcomes for a specific case. But this requires data to be collected across multiple years with certain conditions for the model to be able to predict outcomes.
Treatment – While currently Medical practitioners use some data to understand the efficacy and effects of medicines, there is a limit to that and importantly it’s not possible to use some past data at a detailed level to come out with custom prescription for each patient. But with AI, it is possible to track the progress of a patient treatment based on the existing conditions, medication prescribed and use that for future patients. This also requires data that needs to be tracked over multiple years before being put to use.
One such use case is treatment of cancer tumors. When biopsy is done, a small portion of the tissue is analyzed and these samples are stored. The images of these tissues can be given to an AI algorithm along with multiple other parameters like demographics of the patient, diagnosis, treatment given, response and various such parameters. Using all this data, the AI model will be able to predict what kind of a treatment would be suitable given an image of a cancerous sample. But as mentioned this takes time and importantly large amount of data is required.
Diagnosis – This is one area where AI can have an immediate and perceptible impact. In this post we shall look at few use cases where usage of AI can significantly help in diagnosing conditions.
Detection of TB based on Chest X Rays
Tuberculosis (TB) is still a pretty fatal disease in developing nations like India, Africa etc. More than 80% of the TB cases are pulmonary TB cases. And pulmonary TB can be easily detected in chest X Rays. The primary reason for TB fatality is not because there is no treatment, but because of lack of timely diagnosis. There are TB hotspot areas in various states of the country where TB is quite prevalent. These being predominantly rural areas, there is no proper access to X Ray/Diagnostic centers. Importantly, access to a radiologist is much restricted. India has a radiologist for every 1 lakh of population, whereas US has 1 radiologist for 10000 of its population.
Given the above situation, if a mobile van can be constituted with a X Ray machine connected to a laptop, which can run the inference software, then this van can be taken to the TB hotspot areas. Suspected patients can be run through the X Ray and within seconds, the software will be able to say whether the patient is TB +ve or -ve. Those suspected patients can then go to a PHC or a hospital in a nearby town to get the treatment. Obviously, the software should be properly calibrated to ensure false negatives are close to 0. This way AI can save lives.
Remote monitoring for chronic patients
It’s very expensive for chronic patients to stay in a hospital for longer periods, especially for monitoring. Importantly for old patients this has to be a regular process. So, there are devices that can monitor multiple critical parameters and vitals of a patient and these devices can be used at home. This data from these devices can be sent to a central server (at a hospital) continuously, which can be analyzed for abnormalities and the relevant stakeholders (like doctors, Medical practitioners etc) can be immediately notified so that a prompt action can be taken. This will definitely save the human lives. This kind of a system can be useful for post-surgery monitoring as well, instead of the patients visiting the hospitals periodically for routine checkups.
Emergency Room
There are multiple use cases that AI can solve in an ER. But lets discuss one use case – Brain stroke. When a patient enters an ER with a brain stroke, its very important to determine what kind of a stroke it is – Hemorrhage or Ischemic. This can be determined through a CT Scan. And this inference can be made by an expert radiologist. The type of stroke detection is important, as the treatments for each of these types are exactly opposite. For hemorrhagic strokes, you don’t give blood thinners, whereas for Ischemic strokes you give blood thinners. Given the limited access to an expert radiologist, an AI software can immediately detect the type of stroke from the CT films and appropriate medication can be immediately administered. Apparently (American Heart association Study) for every minute of delayed treatment, around 2 million neurons are lost in the brain. So, time saved is brain saved. The future is extremely bright for AI being an integral part of Healthcare and importantly without any bias.